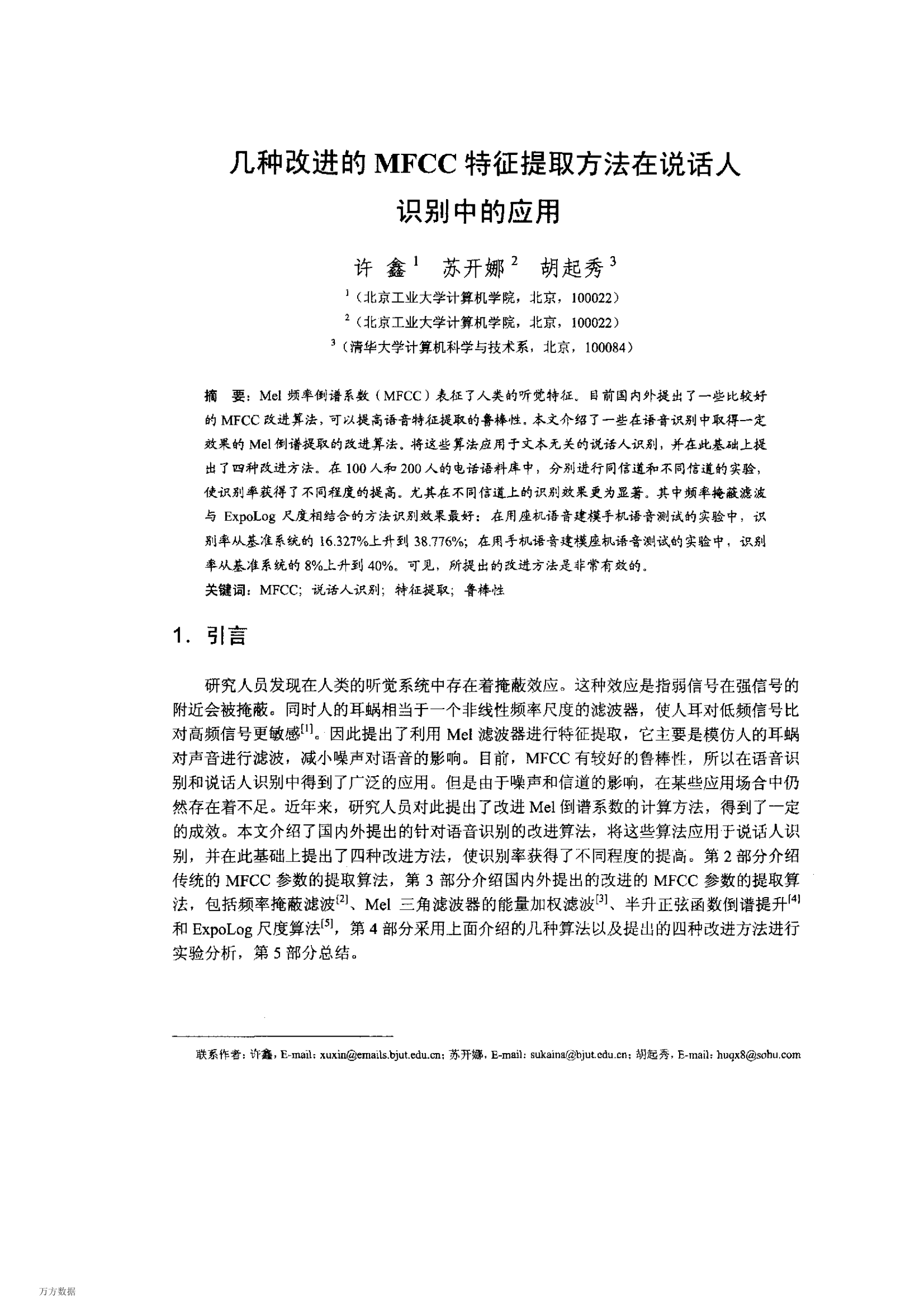
第1页 / 共7页
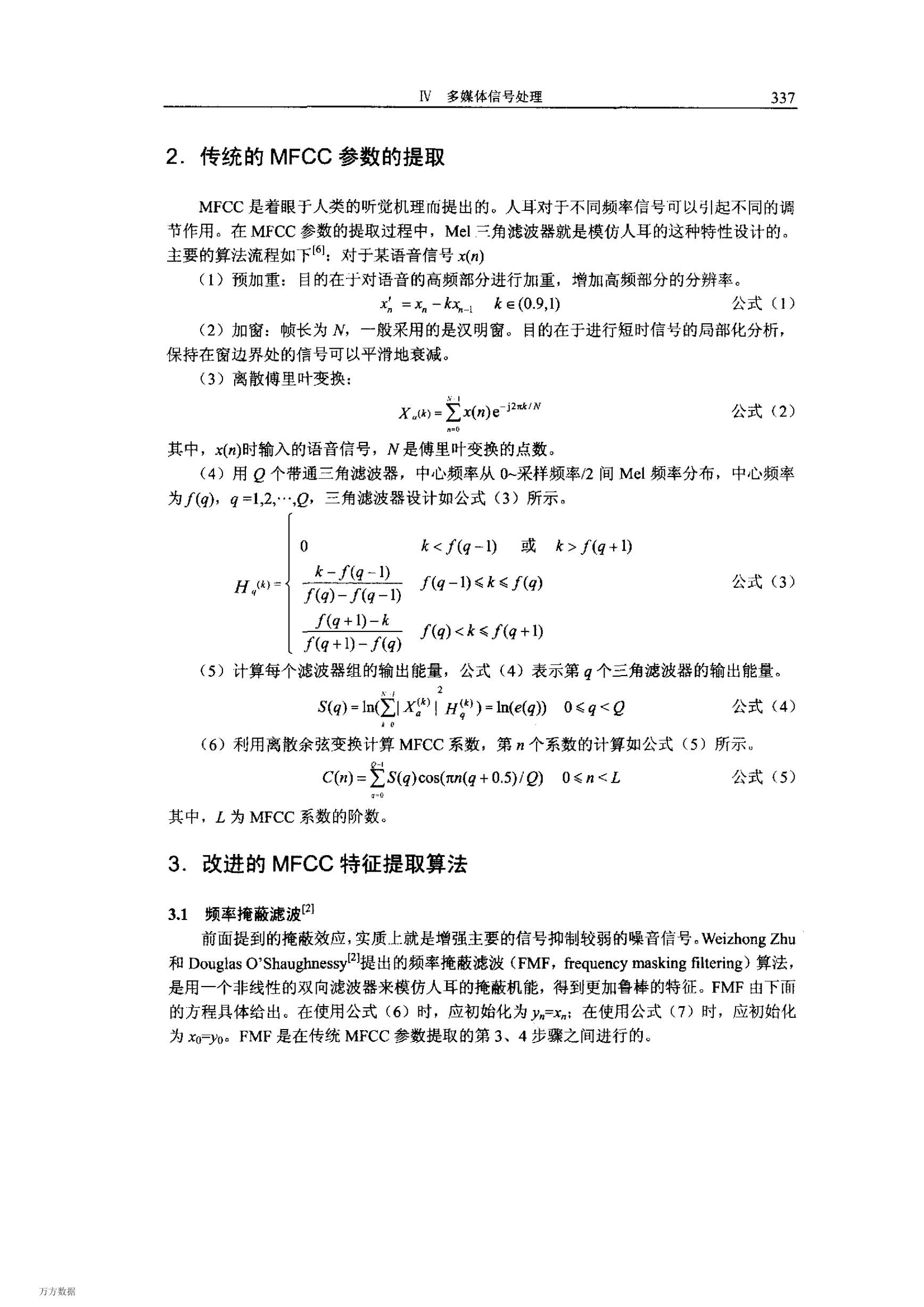
第2页 / 共7页
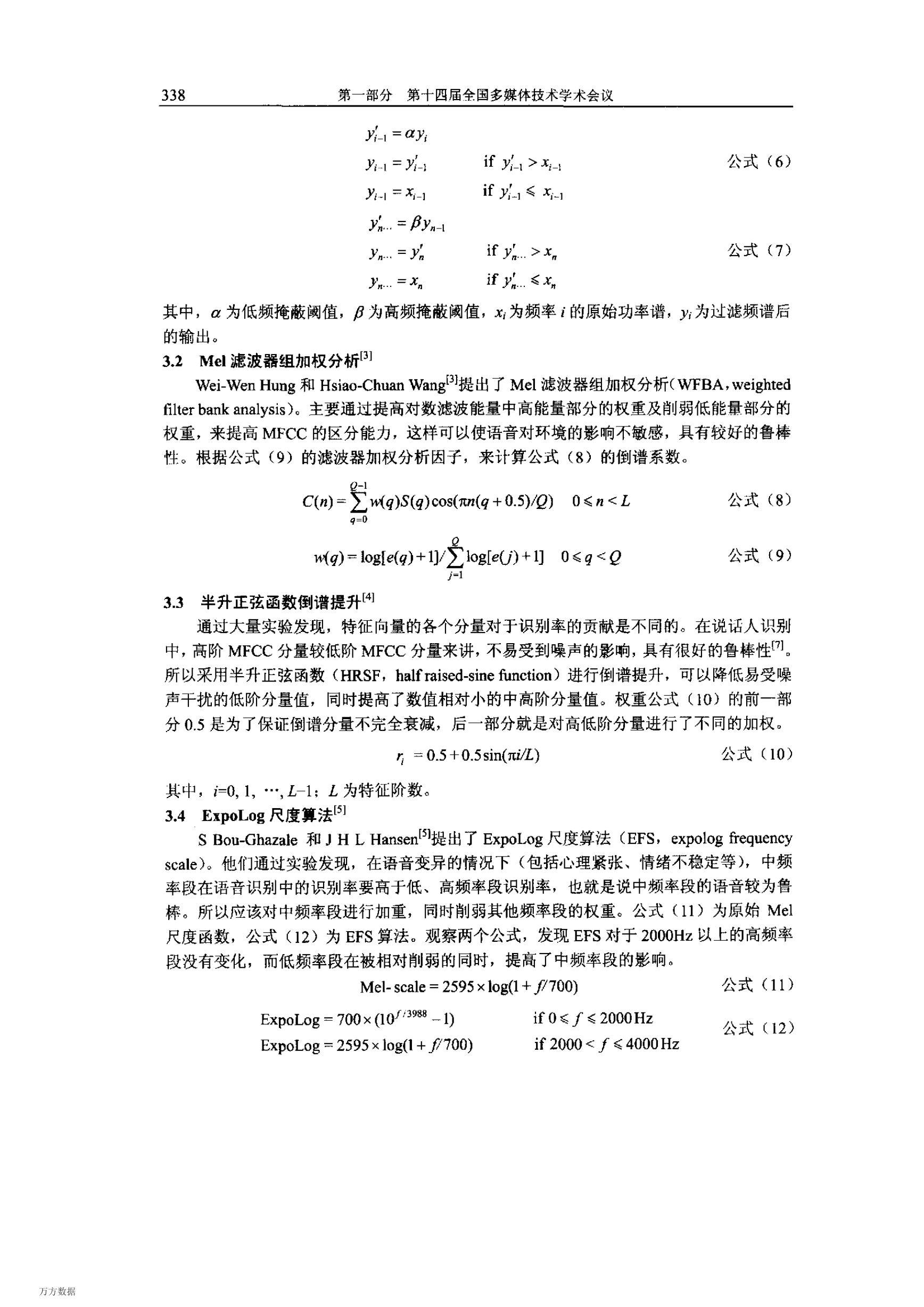
第3页 / 共7页
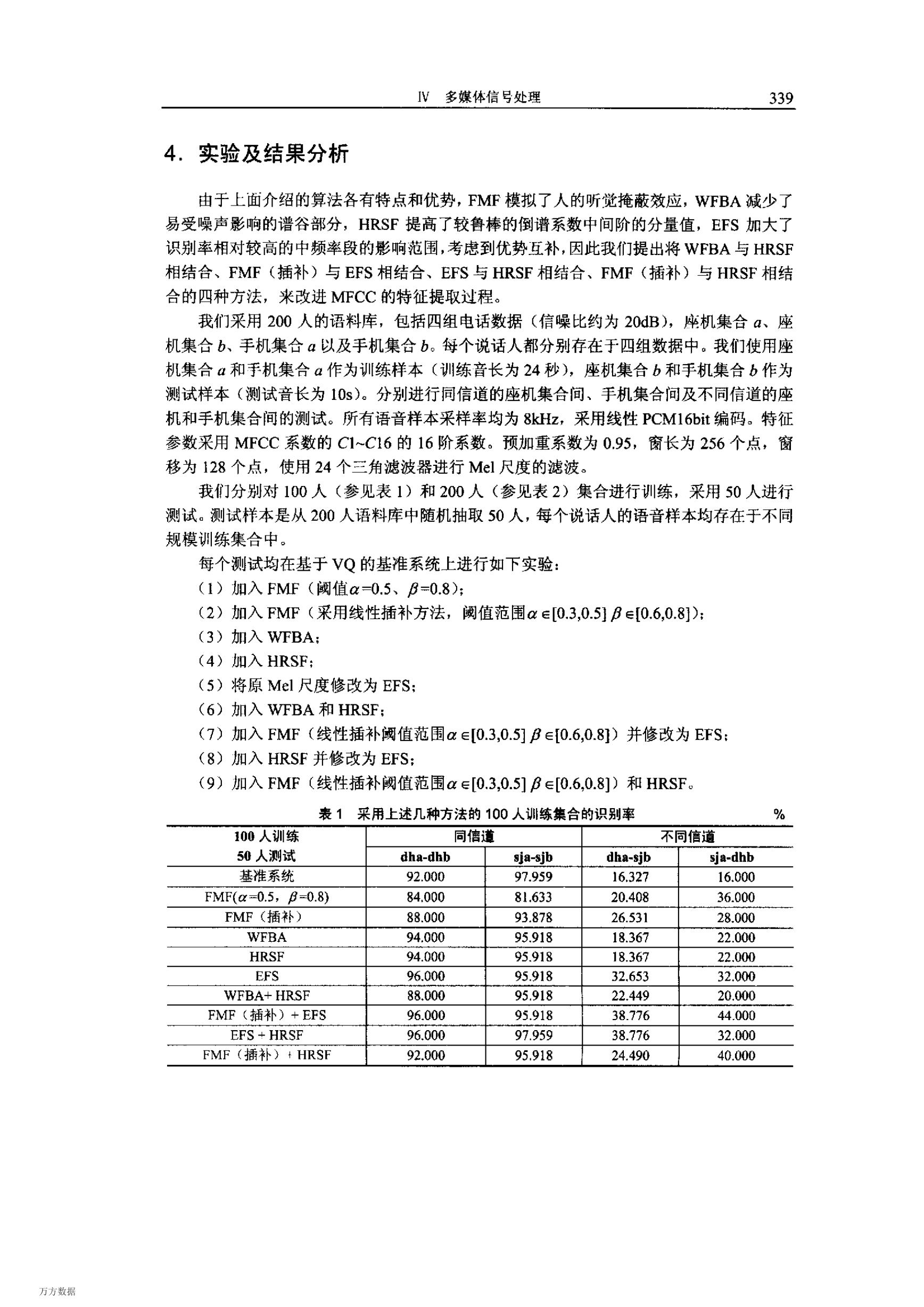
第4页 / 共7页
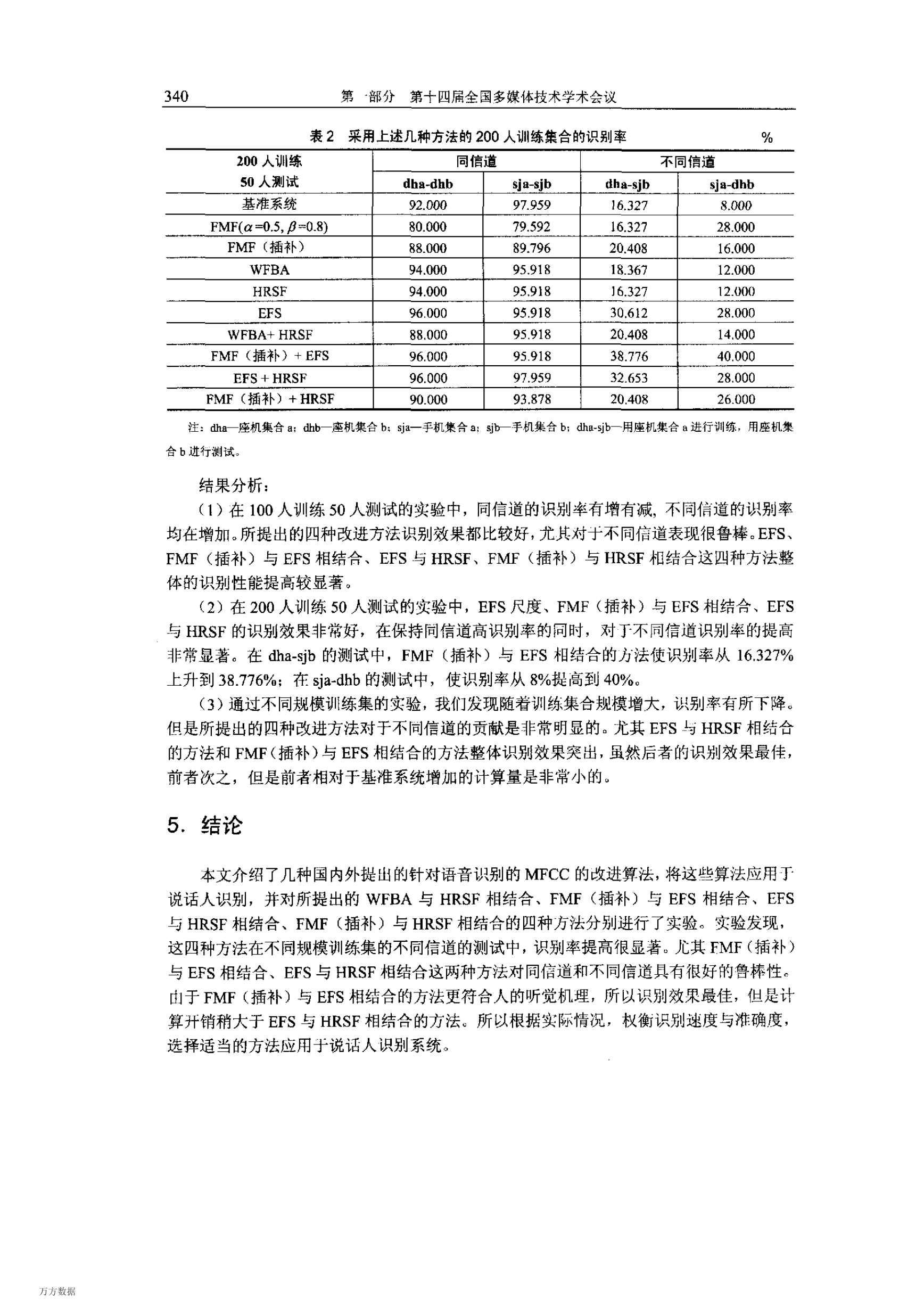
第5页 / 共7页
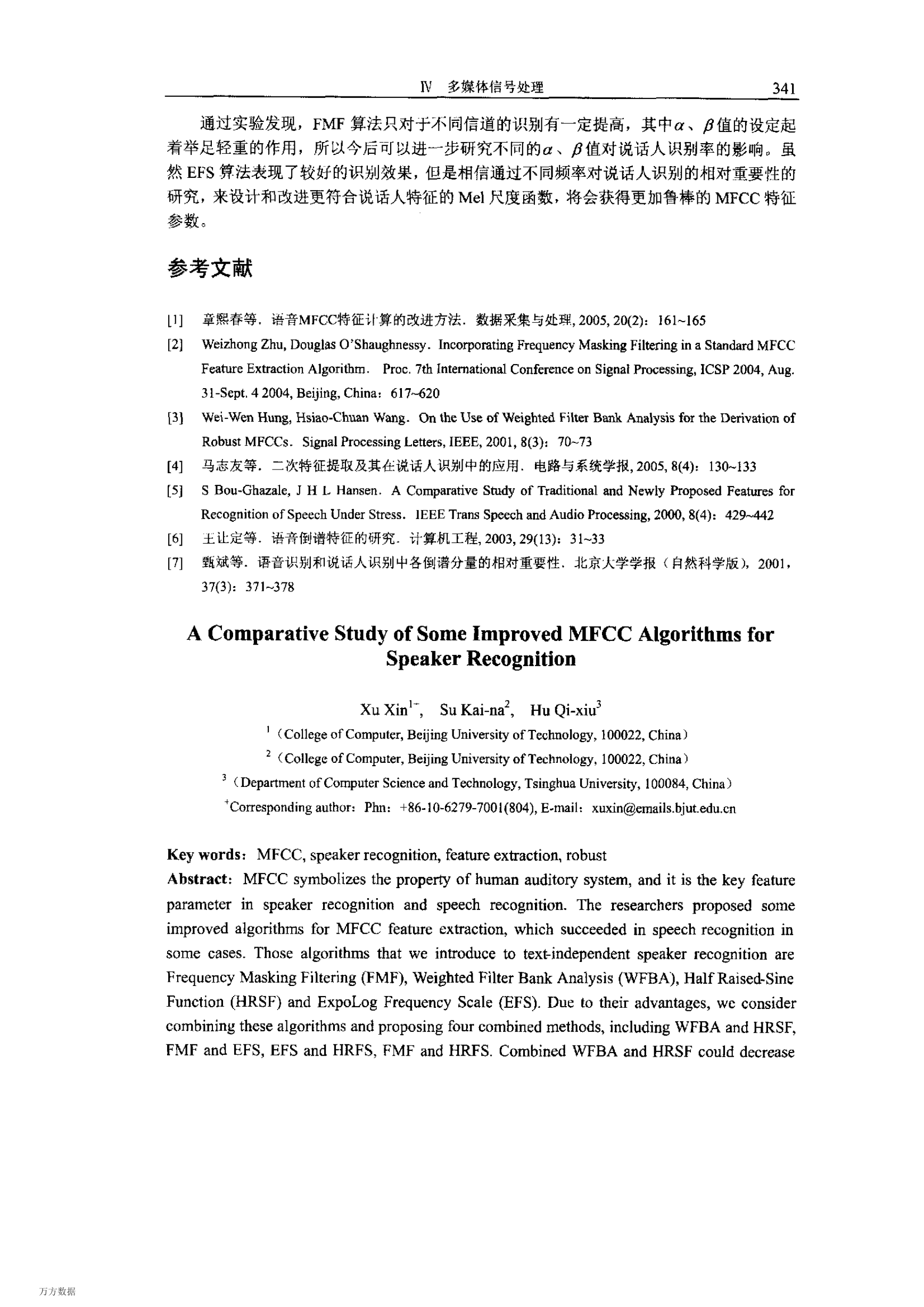
第6页 / 共7页
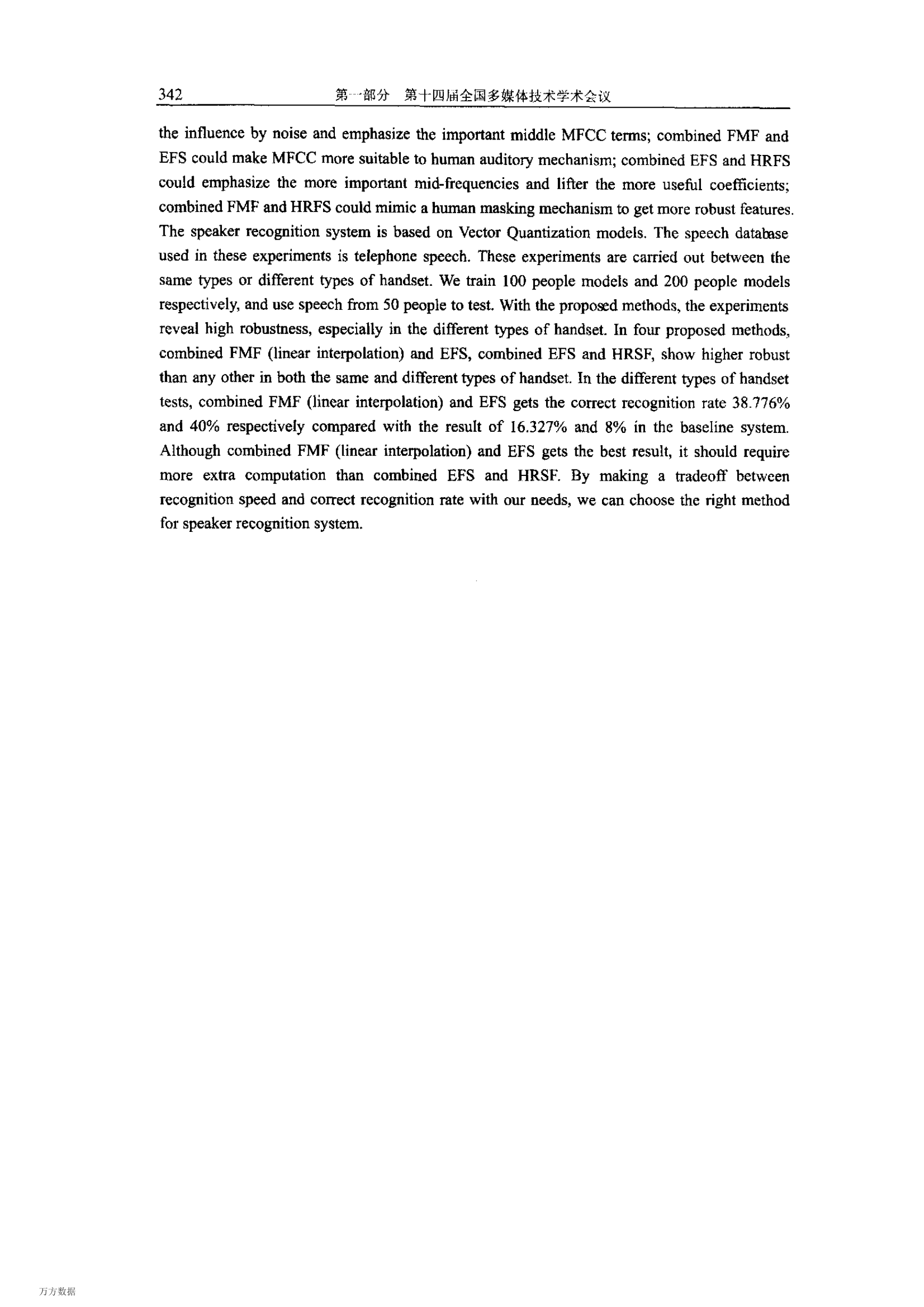
第7页 / 共7页
IV 多媒体信 号处理 339 4.实验及结果分析 由于上面介绍的算法各有特点和优势,FMF模拟了人的听觉掩蔽效应,WFBA减少了 易受噪声影响的谱谷部分,HRSF提高了较鲁棒的倒谱系数中间阶的分量值,EFS加大了 识别率相对较高的中频率段的影响范围,考虑到优势互补,因此我们提出将 WFBA与HRSF 相结合、FMF(插补)与EFS相结合、EFS与 HRSF相结合、FMF(插补)与 HRSF相结 合的四种方法,来改进MFCC的特征提取过程。 我们采用 200 人的语料库,包括四组电话数据 (信噪比约为20dB ),座机集合 a、座 机集合 b、手机集合a以及手机集合 b。每个说话人都分别存在于四组数据中。我们使用座 机集合a和手机集合a作为训练样本 〔训练音长为24 秒),座机集合b和手机集合b作为 测试样本 〔测试音长为 IDs)。分别进行同信道的座机集合间、手机集合间及不同信道的座 机和手机集合间的测试。所有语音样本采样率均为skHz,采用线性PcM16bit编码。特征 参数采用 MFCC系数的 CI一C16的 16阶系数。预加重系数为 。.95,窗长为 256 个点,窗 移为 128个点,使用 24 个三角滤波器进行 Mel尺度的滤波。 我们分别对 100人 (参见表 1) 和 200人 (参见表 2) 集合进行训练,采用 50 人进行 测试 测试样本是从 200 人语料库中随机抽取 50 人,每个说话人的语音样本均存在于不同 规模训练集合中。 每个测试均在基于vQ的基准系统上进行如下实验: (1)加入F‘】「(闭值a=0.5、刀=0名); (2)加入FMF(采用线性插补方法,闭值范围a以。入住5]刀以。衣众8〕); (3)加入 WFBA; (4)加入 HRSF; (5)将原Mel尺度修改为EFS; (6)加入 WFBA和 HRSF; (7) 加入FMF(线性插补阂值范围a以0.3几5]夕姚a6瓜81)并修改为EFS; (8)加入 HRSF并修改为 EFS; (9)加入FMF(线性插补阂值范围a。[03,0乃]刀。[0石,0名])和HRsF 表 1 采用上述几种方法的 100人训练集合的识别率 % 同信道 一} 不同信道 100人训练 50 人测试 基准系统 FMF(插补) dha_dhb 一} sja习b 一} dh卜sjb {} 习a.dhb } 92刀00 1} 97,5, 一} 16o27 }} 16.000 FMF(a一0石,夕一0名) } 54刀00 一} 51.633 一} 20.408 一} 36000 } 58.000 93.575 一} 26531 一} 25一000 } 94刀00 一1 95.915 一} 18.367 一} 22.000 } 94000 一1 95,15 一} 15.367 } 96.000 95夕18 32石53 88刀00 } 95.915 22t449 1 20000 96力00 } 95.915 38776 } 440。。 96刀00 } 97959 } 35.776 } 3200。 92.000 } 951918 40.00 0 FMF(插补 )+EFS FMF(插补)十HRS「 W FBA+HR名F W FBA HRSF EFS 22.000 32刀00 EFS山HRSF 24.490 万方数据340 第 一部分 第+四届全国多媒体技术学术会议 表2 采用上述几种方法的200人训练集合的识别率 200人训练 50人测试 基准系统 同信道 } dha-dhb } sja-sjb dha-sjb 92.000 97.959 16.327 FMF(a=0.5,刀=0.8) } 80.000 一} } 88.000 } 89796 } 不同信道 sja-dhb 8.OO0 28.000 } FMF(插补) W FBA HRSF EFS 94.000 94.000 } W FBA+ HRSF 88.000 FMF(插补)十EFS } } } } FMF (插补 )+HRSF } ,。。。。 } EFS + HRSF 95.918 } 18.367 } 12.000 95.918 } } 一} 95.918 95.918 20.408 } 38.776 32.653 } 14.000 40.000 28.000 26.000 注:dhs 座机集合。;dhb 座机集合b: sja-手机集合a; sjb,一手机集合b; dha-sjb一用座机集合。进行训练,用座机集 合 b进行测试 结果分析: (1)在 100人训练50人钡(试的实验中,同信道的识别率有增有减,不同信道的识别率 均在增加。所提出的四种改进方法识别效果都比较好,尤其对十不同信道表现很鲁棒.EFS. FMF(插补)与 EFS相结合、EFS与HRSF, FMF(插补)与 HRSF相结合这四种方法整 体的识别性能提高较显著。 (2)在200人训练50人测试的实验中,EFS尺度、FMF(插补)与EFS相结合、EFS 与HRSF的识别效果非常好,在保持同信道高识别率的同时,对于不同信道识别率的提高 非常显著 在 dha-sjb的测试中,FMF(插补)与 EFS相结合的力法使识别率从 16.327% 上升到38.776%;在sja-dbb的测试中,使识别率从8%提高到40%0 (3)通过不同规模训练集的实验,我们发现随着训练集合规模增大,识别率有所下降 但是所提出的四种改进方法对于不同信道的贡献是非常明显的。尤其EFS与HRSF相结合 的方法和 FMF(插补)与EFS相结合的方法整体识别效果突出,虽然后者的识别效果最佳, 前者次之,但是前者相对于基准系统增加的计算量是非常小的。 5.结论 本文介绍了几种国内外提出的针对语音识别的MFCC的改进算法,将这些算法应用于 说话人识别,并对所提出的 WFBA与 HRSF相结合、FMF(插补)与 EFS相结合、EFS 与HRSF相结合、FMF(插补)与HRSF相结合的四种方法分别进行了实验。实验发现, 这四种方法在不同规模训练集的不同信道的测试中,识别率提高很显著。尤其FMF(插补) 与EFS相结合、EFS与HRSF相结合这两种方法对同信道和不同信道具有很好的鲁棒性 山于FMF(插补)与EFS相结合的方法更符合人的听觉机理,所以识别效果最佳,但是计 算开销稍大于EFS与HRSF相结合的方法。所以根据实际情况,权衡识别速度与准确度, 选择适当的方法应用于说话人识别系统。 万方数据>t1 多媒体信 号处理 341 通过实验发现,FMF算法只对于不同信道的识别有一定提高,其中a,刀值的设定起 着举足轻重的作用,所以今后可以进一步研究不同的a, '6值对说话人识别率的影响 虽 然EFS算法表现了较好的识别效果,但是相信通过不同频率对说话人识别的相对重要性的 研究,来设计和改进更符合说话人特征的Mel尺度函数,将会获得更加鲁棒的MFCC特征 参数。 参考文献 []j [2j [3] 14] [5j [6j [7] 章熙春等t语音MFCC特征计算的改进方法.数据采集与处理,2005,20(2): 161-165 Weizhong Zhu, Douglas O'Shaughnessy. Incorporating Frequency Masking Filtering in a Standard MFCC Feature Extraction Algorithm. Proc. 7th International Conference on Signal Processing, ICSP 2004, Aug. 31-Sept. 4 2004, Beijing, China: 617--620 Wei-Wen Hung, Hsiao-Chuan Wang. On the Use of Weighted Filter Bank Analysis for the Derivation of Robust MFCCs. Signal Processing Letters, IEEE, 2001, 8(3): 70-73 马志友等.二次特征提取及其在说话人识别中的应用.电路与系统学报,2005,8(4): 130-133 S Bou-Ghazale, S H L Hansen. A Comparative Study of Traditional and Newly Proposed Features for Recognition of Speech Under Stress. IEEE Trans Speech and Audio Processing, 2000, 8(4): 429-442 土让定等.语音倒谱特征的研究一计算机工程,2003, 29(13): 31-33 甄斌等.语音识别和说话人识别中各倒谱分量的相对重要性.北京大学学报 (自然科学版),2001, 37(3): 371-378 A Comparative Study of Some Improved MFCC Algorithms for Speaker Recognition Xu Xin,一,Su Kai-naz, Hu Qi-xiu3 (College of Computer, Beijing University ofTechnology, 100022, China) 1 ' (College of Computer, Beijing University of Technology, 100022, China) ' (Department of Computer Science and Technology, Tsinghua University, 100084, China) 'Corresponding author: Phn:十86-10-6279-7001(804),E-mail: xuxin@emails.bjut.edu.cn Key words: MFCC, speaker recognition, feature extraction, robust Abstract: MFCC symbolizes the property of human auditory system, and it is the key feature parameter in speaker recognition and speech recognition. The researchers proposed some improved algorithms for MFCC feature extraction, which succeeded in speech recognition in some cases. Those algorithms that we introduce to text-independent speaker recognition are Frequency Masking Filtering (FMF), Weighted Filter Bank Analysis (WFBA), Half Raised-Sine Function (HRSF) and ExpoLog Frequency Scale (EFS). Due to their advantages, we consider combining these algorithms and proposing four combined methods, including WFBA and HRSF, FMF and EFS, EFS and HRFS, FMF and HRFS. Combined WFBA and HRSF could decrease 万方数据342 第 部分 第十四届全国多媒体技术学术会议 the influence by noise and emphasize the important middle MFCC terms; combined FMF and EFS could make MFCC more suitable to human auditory mechanism; combined EFS and HRFS could emphasize the more important mid-frequencies and lifter the more useful coefficients; combined FMF and HRFS could mimic a human masking mechanism to get more robust features The speaker recognition system is based on Vector Quantization models. The speech database used in these experiments is telephone speech. These experiments are carried out between the same types or different types of handset. We train 100 people models and 200 people models respectively, and use speech from 50 people to test. With the proposed methods, the experiments reveal high robustness, especially in the different types of handset. In four proposed methods, combined FMF (linear interpolation) and EFS, combined EFS and HRSF, show higher robust than any other in both the same and different types of handset. In the different types of handset tests, combined FMF (linear interpolation) and EFS gets the correct recognition rate 38.776% and 40% respectively compared with the result of 16.327% and 8% in the baseline system Although combined FMF (linear interpolation) and EFS gets the best result, it should require more extra computation than combined EFS and HRSF. By making a tradeoff between recognition speed and correct recognition rate with our needs, we can choose the right method for speaker recognition system. 万方数据